Understanding Cluster Sampling: A Comprehensive Overview
Jessie A Ellis Jun 10, 2024 08:08
Cluster sampling divides a population into smaller clusters, simplifying large-scale research.
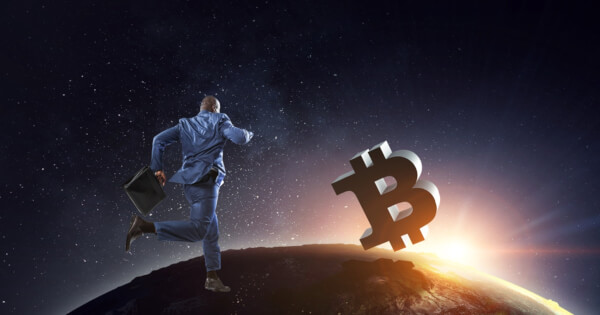
Cluster sampling is a probability sampling method where researchers divide a population into smaller groups called clusters. They then form a sample by randomly selecting clusters, according to Quillbot Blog.
What Is Cluster Sampling?
The most basic form of cluster sampling is single-stage cluster sampling, which consists of four steps:
Step 1: Determine the Population
The first step for every sampling method is defining the population you are interested in. For example, if you want to study eighth-graders, you would list all schools and then randomly select some of these schools.
Step 2: Divide the Population into Clusters
The quality of the clusters significantly influences the validity of the results. Ideal clusters should be as diverse as possible and collectively represent the entire population without any overlap between clusters. Homogeneous clusters (e.g., only Christian schools) may introduce biases.
Step 3: Conduct Random Sampling to Select Clusters
Randomly selecting clusters ensures that each cluster represents the population, thereby increasing the validity of the results. Even if clusters do not perfectly represent the population, random sampling still provides an overview of the entire population.
Step 4: Collect Data from Your Sample
Researchers then proceed to conduct their research and collect data from the selected clusters. The number of clusters chosen depends on the required sample size, which is determined by the population size, chosen confidence level, confidence interval, and estimated standard deviation.
Stratified vs. Cluster Sampling
Though similar, stratified sampling and cluster sampling have distinct differences. In stratified sampling, the population is divided into strata based on specific traits (e.g., age), and members are randomly selected from each stratum. Each stratum is not a mini-version of the population. In cluster sampling, the population is divided into naturally occurring clusters (e.g., neighborhoods), and the entire cluster is sampled without requiring participants to meet specific criteria. Each cluster is a mini-version of the population.
What Is Multistage Cluster Sampling?
Multistage cluster sampling involves additional random sampling within the selected clusters, known as double-stage sampling. This method is useful when single-stage cluster sampling is too costly or time-consuming. Researchers can further repeat the sampling process, known as multistage sampling, which narrows down the sample size, making data collection more manageable.
Advantages and Disadvantages of Cluster Sampling
Cluster sampling has several advantages:
- It is inexpensive and efficient, especially for large geographic areas.
- It ensures high external validity if the population is appropriately clustered.
However, it also has some disadvantages:
- Internal validity is lower compared to single random sampling, especially in multistage sampling.
- Accurate representation of the population is harder, potentially leading to biased results.
- Cluster sampling requires thorough preparation and is often more complex than other sampling methods.
Frequently Asked Questions about Cluster Sampling
What are the different types of cluster sampling?
In all types of cluster sampling, the population is divided into clusters before drawing a random sample. The subsequent steps depend on the specific type of cluster sampling:
- Single-stage cluster sampling: Collect data from every unit in the selected clusters.
- Double-stage cluster sampling: Draw a random sample of units within the clusters and collect data from this sample.
- Multistage cluster sampling: Repeat the random sampling process within the clusters until a sufficiently small sample is obtained.
What are the disadvantages of cluster sampling?
Cluster sampling often harms internal validity, especially with multiple clustering stages. Results are more likely to be biased and invalid, particularly if clusters do not accurately represent the population. Additionally, cluster sampling is generally more complex than other sampling methods.
Image source: Shutterstock