NVIDIA Introduces Hydra-MDP for Scalable End-to-End Autonomous Driving
Caroline Bishop Jun 18, 2024 06:45
NVIDIA unveils Hydra-MDP, a cutting-edge framework for end-to-end autonomous driving, enhancing scalability and safety through multi-teacher knowledge distillation.
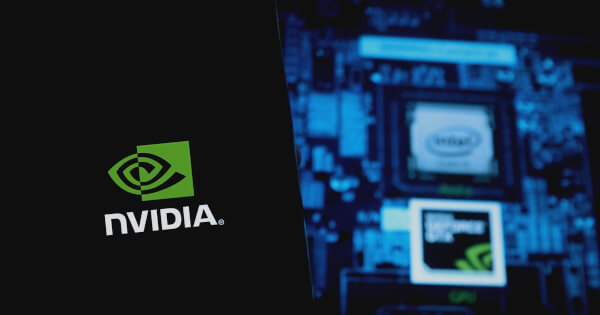
NVIDIA has unveiled Hydra-MDP, an innovative framework designed to advance the field of end-to-end autonomous driving. According to the NVIDIA Technical Blog, Hydra-MDP leverages a multi-teacher, student-teacher knowledge distillation architecture to enhance the scalability and safety of autonomous driving systems.
Groundbreaking Framework for Autonomous Driving
Building an autonomous system that efficiently navigates complex environments while ensuring passenger safety and comfort is a significant challenge. Hydra-MDP addresses this by integrating knowledge from both human and rule-based planners, allowing the model to learn diverse trajectories and improve generalization across various driving scenarios.
This universal framework showcases how machine learning-based planning can be bolstered by rule-based planners, ensuring that the model not only mimics human driving behaviors but also adheres to traffic rules and safety standards. This integration addresses the limitations of traditional imitation learning techniques.
Data-Driven Scalability and Robustness
Hydra-MDP’s data-driven scaling laws underscore its robustness and adaptability. By utilizing pretrained foundation models with extensive datasets and GPU hours, Hydra-MDP demonstrates its potential for continuous improvement. The framework’s scalability was highlighted when it won first place and the innovation award at the E2E Driving at Scale Challenge at CVPR 2024, outperforming state-of-the-art planners on the nuPlan benchmark.
Key Lessons and Innovations
Hydra-MDP’s development has provided several critical insights that shaped its architecture and success:
- Embrace Multimodal and Multi-Target Planning: Traditional systems often focus on single-modal and single-target objectives, limiting their real-world effectiveness. Hydra-MDP integrates diverse trajectories tailored to multiple metrics, including safety, efficiency, and comfort.
- Utilize Multi-Target Hydra-Distillation: By employing multiple specialized teachers (both human and rule-based), the model predicts trajectories that align with various simulation-based metrics, enhancing generalization across diverse driving conditions.
- Overcome Post-Processing Limitations: Hydra-MDP integrates perception and planning into a seamless pipeline, maintaining the richness of environmental data throughout the decision-making process.
- Incorporate Environmental Context: Using features from LiDAR and camera inputs, Hydra-MDP’s perception network better understands and reacts to complex driving environments.
- Iterative Refinement through Simulation: Extensive offline simulations generate ground truth simulation scores, which supervise the training process, bridging the gap between theoretical performance and real-world applicability.
- Effective Model Ensembling: Techniques like Mixture of Encoders and Sub-score Ensembling combine model strengths, improving robustness and handling a diverse array of driving scenarios with high accuracy.
Conclusion
The development of Hydra-MDP represents a significant leap forward in autonomous driving technology. By embracing multimodal and multi-target planning, leveraging multi-target hydra-distillation, and refining through extensive simulations, NVIDIA has created a model that significantly outperforms existing state-of-the-art methods. These advancements offer a promising roadmap for the future of AI-driven autonomous systems.
For more information, see the full details on the NVIDIA Technical Blog.
Image source: Shutterstock