Evaluating AI Systems: The Critical Role of Objective Benchmarks
Lawrence Jengar Aug 06, 2024 02:44
Learn how objective benchmarks are vital for evaluating AI systems fairly, ensuring accurate performance metrics for informed decision-making.
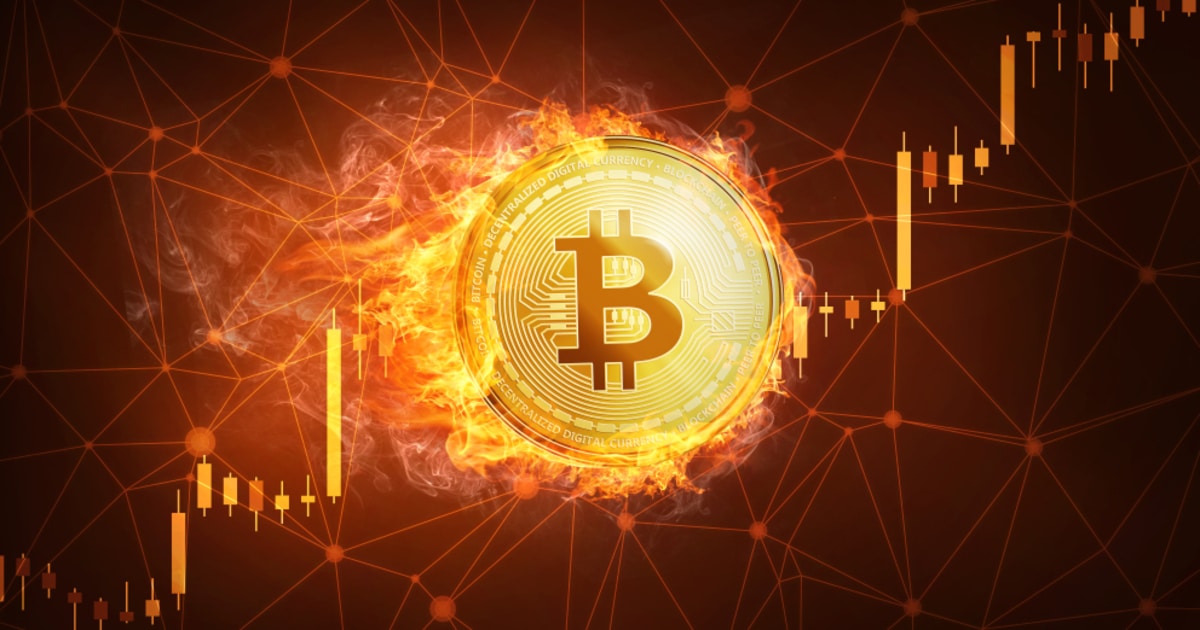
The artificial intelligence industry is projected to become a trillion-dollar market within the next decade, fundamentally altering how people work, learn, and interact with technology, according to AssemblyAI. As AI technology continues to evolve, there is an increasing need for objective benchmarks to fairly evaluate AI systems and ensure that they meet real-world performance standards.
The Importance of Objective Benchmarks
Objective benchmarks provide a standardized, unbiased method to compare different AI models. This transparency helps users understand the capabilities of various AI solutions, fostering informed decision-making. Without consistent benchmarks, evaluators risk obtaining skewed results, leading to suboptimal choices and poor user experiences. AssemblyAI emphasizes that benchmarks validate the performance of AI systems, ensuring they can solve real-world problems effectively.
Role of Third-Party Organizations
Third-party organizations play a crucial role in conducting independent evaluations and benchmarks. These organizations ensure assessments are impartial and scientifically rigorous, offering an unbiased comparison of AI technologies. AssemblyAI's CEO, Dylan Fox, highlights the importance of having independent bodies oversee AI benchmarks using open-source datasets to avoid overfitting and ensure accurate evaluations.
According to Luka Chketiani, AssemblyAI's research lead, an objective organization must be competent and impartial, contributing to the growth of the domain by providing truthful evaluation results. These organizations should have no financial or collaborative ties with the AI developers they evaluate, ensuring independence and preventing conflicts of interest.
Challenges in Establishing Third-Party Evaluations
Setting up third-party evaluations is complex and resource-intensive. It requires regular updates to keep pace with the rapidly evolving AI landscape. Sam Flamini, former senior solutions architect at AssemblyAI, notes the difficulty in maintaining benchmarking pipelines due to changing models and API schemas. Additionally, funding is a significant barrier, as expert AI scientists and the necessary computing power require substantial resources.
Despite these challenges, the demand for unbiased third-party evaluations is growing. Flamini anticipates the emergence of organizations that will serve as the "G2" for AI models, providing objective data and continuous evaluations to help users make informed decisions.
Evaluating AI Models: Metrics to Consider
Different applications require different evaluation metrics. For instance, evaluating speech-to-text AI models involves metrics such as Word Error Rate (WER), Character Error Rate (CER), and Real-Time Factor (RTF). Each metric provides insights into specific aspects of the model's performance, helping users choose the best solution for their needs.
For Large Language Models (LLMs), both quantitative and qualitative analyses are essential. Quantitative metrics target specific tasks, while qualitative evaluations involve human assessments to ensure the model's outputs meet real-world standards. Recent research suggests using LLMs to run qualitative evaluations quantitatively, aligning better with human judgment.
Conducting Independent Evaluations
If opting for an independent evaluation, it is crucial to define key performance indicators (KPIs) relevant to your business needs. Setting up a testing framework and A/B testing different models can provide clear insights into their real-world performance. Avoid common pitfalls such as using irrelevant testing data or relying solely on public datasets, which may not reflect practical applications.
In the absence of third-party evaluations, closely examine organizations' self-reported numbers and evaluation methodologies. Transparent and consistent evaluation practices are vital for making informed decisions about AI systems.
AssemblyAI underscores the importance of independent evaluations and standardized methodologies. As AI technology advances, the need for reliable, impartial benchmarks will only grow, driving innovation and accountability in the AI industry. Objective benchmarks empower stakeholders to choose the best AI solutions, fostering meaningful progress in various domains.
Disclaimer: This article focuses on evaluating Speech AI systems and is not a comprehensive guide for all AI systems. Each AI modality, including text, image, and video, has its own evaluation methods.
Image source: Shutterstock