How Meta's FlowVid will Revolutionize Video-to-Video Synthesis with Temporal Consistency
Researchers at Texas at Austin and Meta GenAI developed FlowVid, a V2V synthesis framework that improves synthesis efficiency and quality, outperforming existing models in video creation and editing.
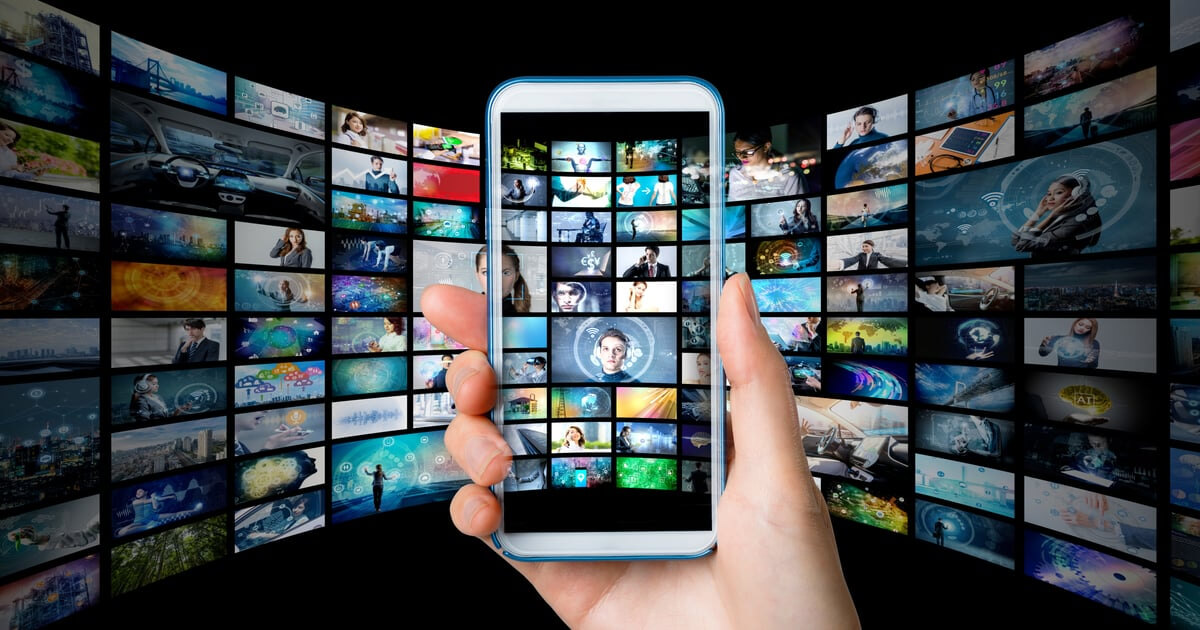
The research paper "FlowVid: Taming Imperfect Optical Flows for Consistent Video-to-Video Synthesis" focuses on addressing the challenges in video-to-video (V2V) synthesis, particularly the issue of maintaining temporal consistency across video frames. This problem is significant in the context of applying image-to-image (I2I) synthesis models to videos, where frame-to-frame pixel flickering often occurs.
The solution proposed in the paper is a new V2V synthesis framework called FlowVid. Developed by researchers from the University of Texas at Austin and Meta GenAI, FlowVid uniquely combines spatial conditions and temporal optical flow clues from the source video. This approach allows for the creation of temporally consistent videos from an input video and a text prompt. The model demonstrates flexibility and efficiency, working seamlessly with existing I2I models to facilitate various modifications such as stylization, object swaps, and local edits.
FlowVid outperforms existing models like CoDeF, Rerender, and TokenFlow in terms of synthesis efficiency. For instance, generating a 4-second video at 30 FPS and 512×512 resolution takes only 1.5 minutes, which is significantly faster than the mentioned models. Additionally, FlowVid ensures high-quality output, as indicated by user studies where it was preferred over other models.
The framework of FlowVid involves training with joint spatial-temporal conditions, employing an edit-propagate procedure for generation. The model allows for editing the first frame using prevalent I2I models and then propagating these edits to successive frames, maintaining consistency and quality.
The researchers conducted extensive experiments and evaluations to demonstrate the effectiveness of FlowVid. These included qualitative and quantitative comparisons with state-of-the-art methods, user studies, and an analysis of the model's runtime efficiency. The results consistently showed that FlowVid offers a robust and efficient approach to V2V synthesis, addressing the longstanding challenge of maintaining temporal consistency in video frames.
For more detailed information and a comprehensive understanding of the methodology and results, the full paper can be accessed at the given URL: https://huggingface.co/papers/2312.17681.
The project's webpage also provides additional insights: https://jeff-liangf.github.io/projects/flowvid/.
Image source: Shutterstock